From Zero to Generative
IAIFI Fellow, MIT
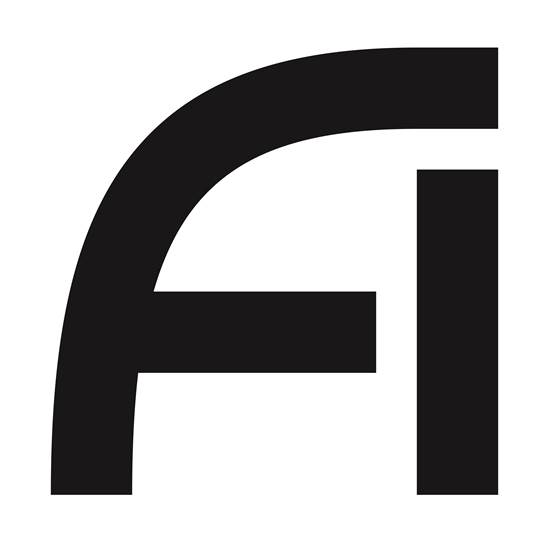
Carolina Cuesta-Lazaro
Art: "The art of painting" by Johannes Vermeer
Learning Generative Modelling from scratch

["Genie 2: A large-scale foundation model" Parker-Holder et al]

["Generative AI for designing and validating easily synthesizable and structurally novel antibiotics" Swanson et al]
Probabilistic ML has made high dimensional inference tractable
1024x1024xTime
Carolina Cuesta-Lazaro IAIFI/MIT - From Zero to Generative

https://parti.research.google
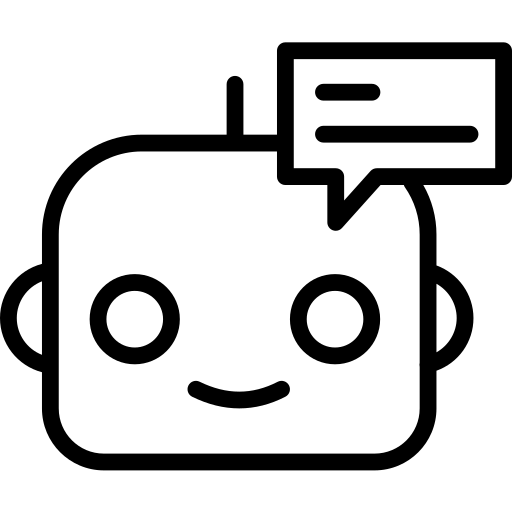
A portrait photo of a kangaroo wearing an orange hoodie and blue sunglasses standing on the grass in front of the Sydney Opera House holding a sign on the chest that says Welcome Friends!



Carolina Cuesta-Lazaro IAIFI/MIT - From Zero to Generative
BEFORE
Artificial General Intelligence?
AFTER

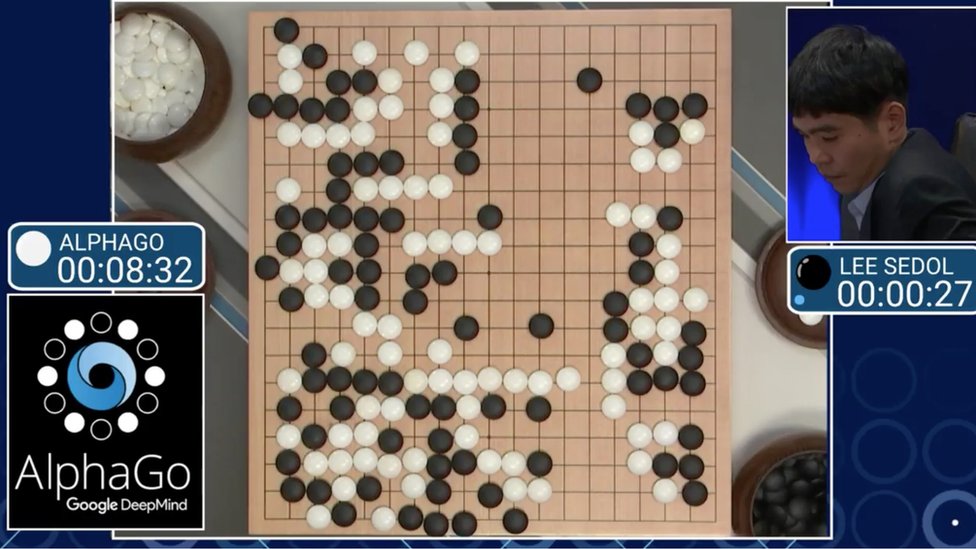
Carolina Cuesta-Lazaro IAIFI/MIT - From Zero to Generative
Scaling laws and emergent abilities
"Scaling Laws for Neural Language Models" Kaplan et al

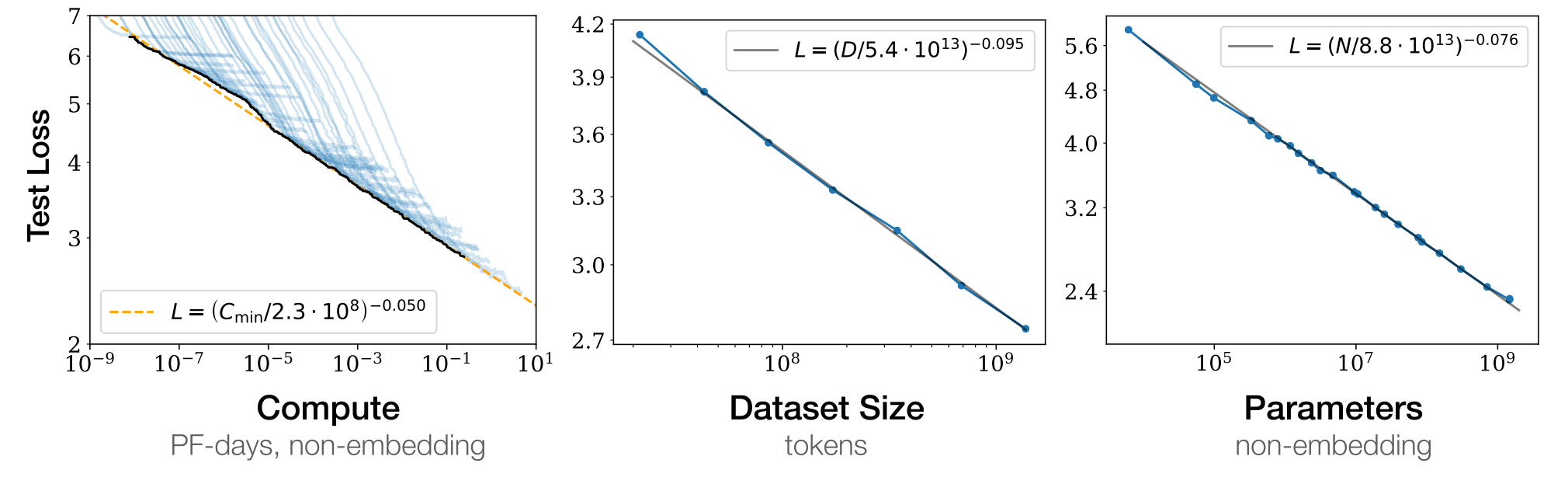
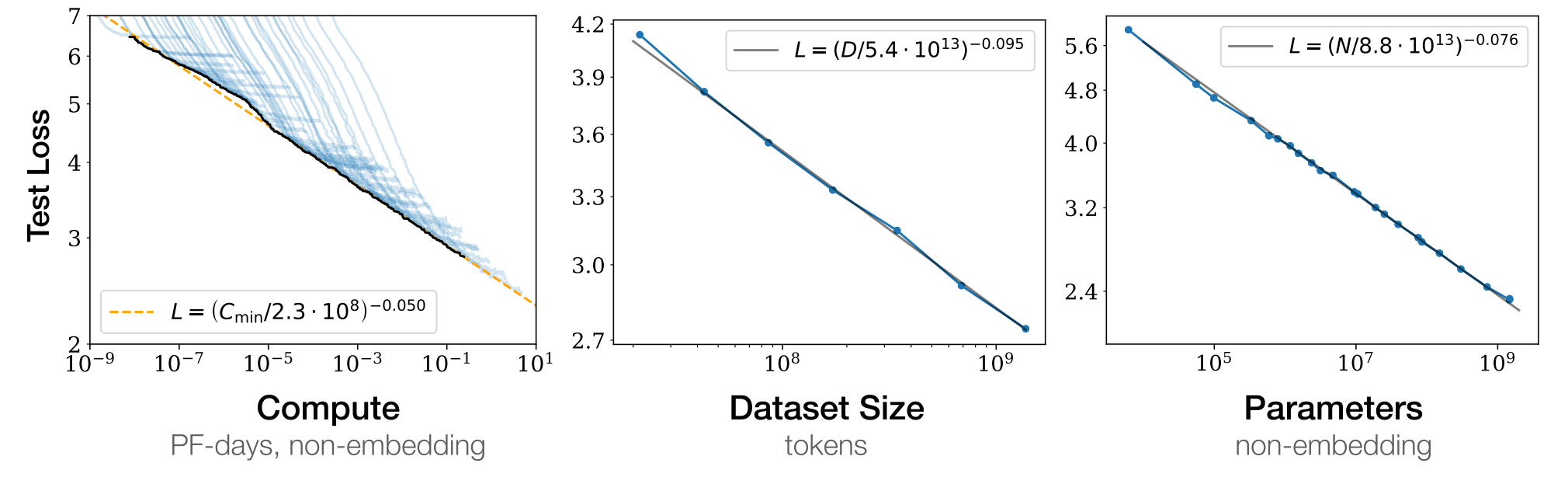
Carolina Cuesta-Lazaro IAIFI/MIT - From Zero to Generative

"Sparks of Artificial General Intelligence: Early experiments with GPT-4" Bubeck et al
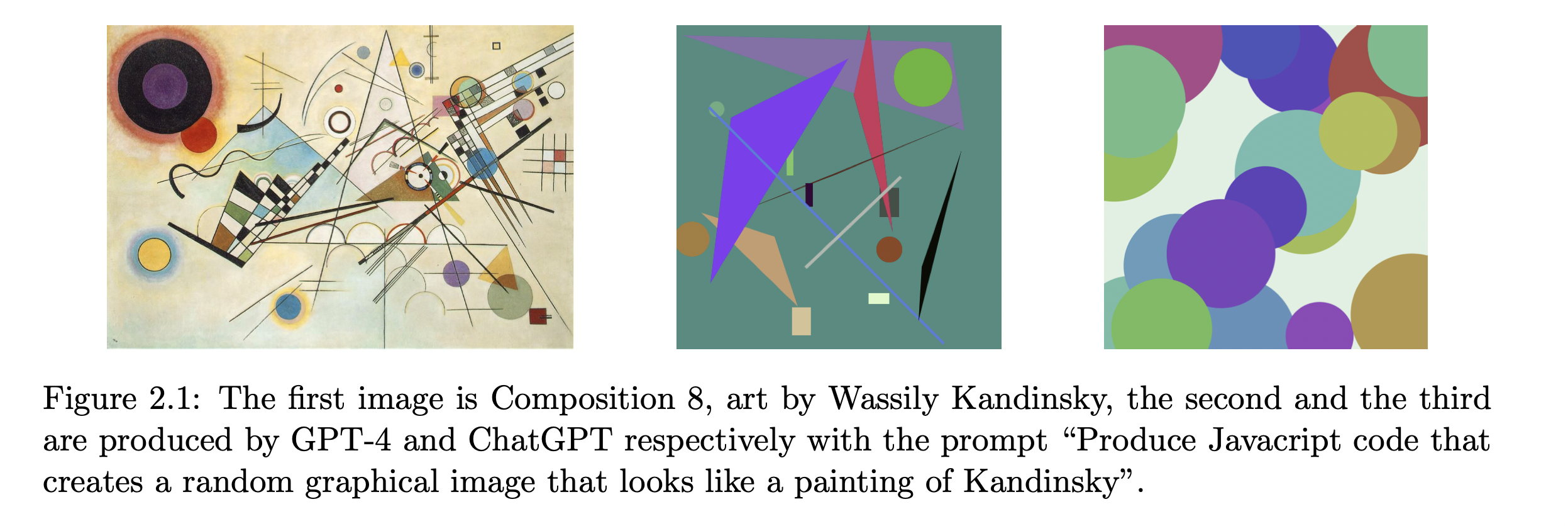
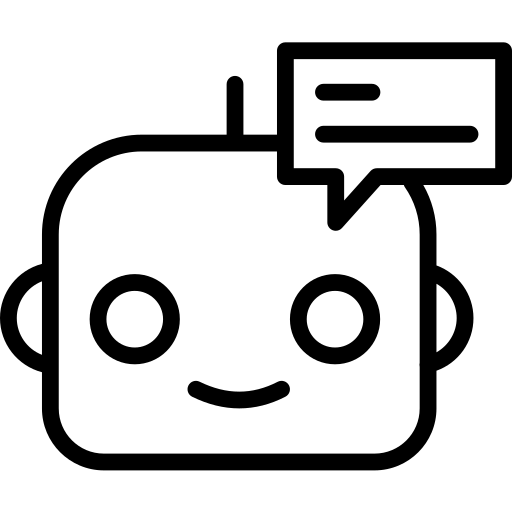
Produce Javascript code that creates a random graphical image that looks like a painting of Kandinsky
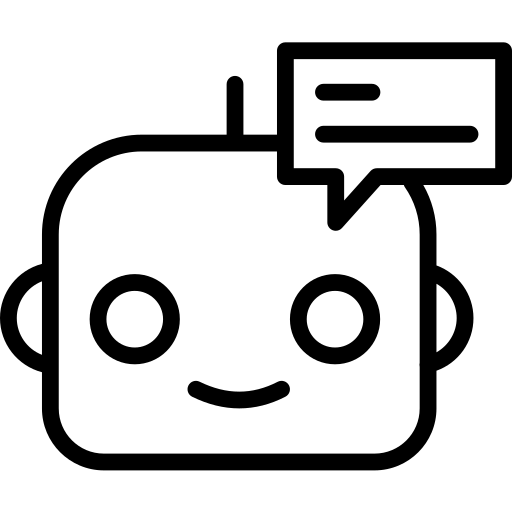
Draw a unicorn in TikZ
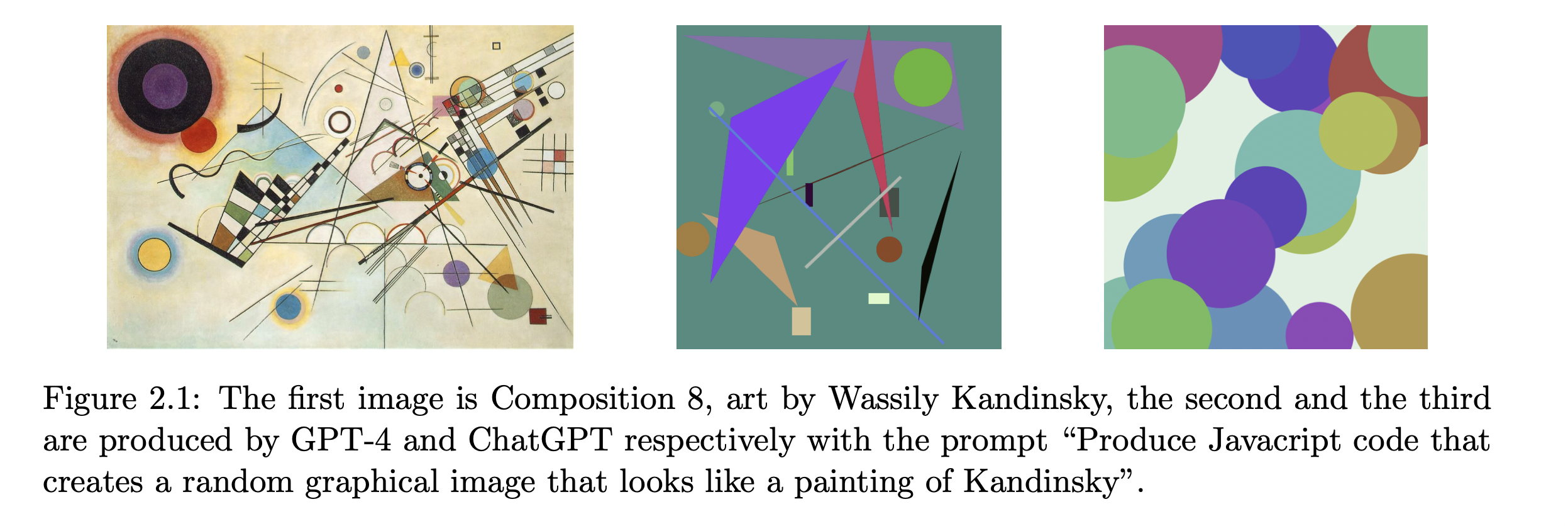
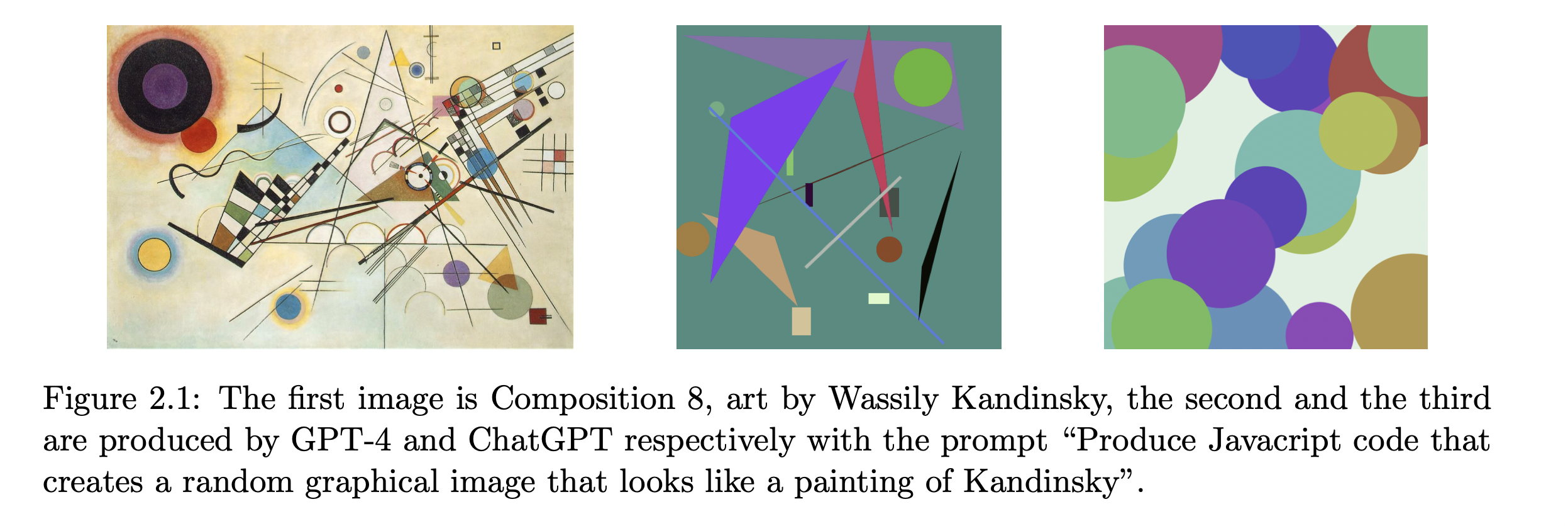


Carolina Cuesta-Lazaro IAIFI/MIT - From Zero to Generative
Today's Plan
1. Machine Learning building blocks
2. Tutorial: Build your first classifier
BREAK
4. Introduction to Generative Models
5. Tutorial: Build your first generative model
Carolina Cuesta-Lazaro IAIFI/MIT - From Zero to Generative
The building blocks: 1. Data
Cosmic Cartography
(Pointclouds)


MNIST
(Images)

Wikipedia
(Text)
Carolina Cuesta-Lazaro IAIFI/MIT - From Zero to Generative

1024x1024
The curse of dimensionality

Inductive biases!
Carolina Cuesta-Lazaro IAIFI/MIT - From Zero to Generative
The building blocks: 2. Architectures

Carolina Cuesta-Lazaro IAIFI/MIT - From Zero to Generative
Image Credit: CS231n Convolutional Neural Networks for Visual Recognition

Pixel 1
Pixel 2
Pixel N

Carolina Cuesta-Lazaro IAIFI/MIT - From Zero to Generative
Multilayer Perceptron (MLP)
Inductive bias: Translation Invariance
Data Representation: Images

Image Credit: Irhum Shakfat "Intuitively Understanding Convolutions for Deep Learning"
Carolina Cuesta-Lazaro IAIFI/MIT - From Zero to Generative
Convolutional Neural Network (CNN)
Inductive bias: Permutation Invariance
Data Representation: Sets, Pointclouds

Carolina Cuesta-Lazaro IAIFI/MIT - From Zero to Generative



Deep Sets


Text
Images
Carolina Cuesta-Lazaro IAIFI/MIT - From Zero to Generative
Transformers
The Unifying architecture?
Inductive bias: Permutation Invariance
Carolina Cuesta-Lazaro IAIFI/MIT - From Zero to Generative
Input token
QUERY: What is X looking for?
KEY: What token X contains
VALUE: What token X will provide
"The dog chased the cat because it was playful."
But, we decide to break permutation invariance!
Positional Encodings
"Dog bites man"
"Man bites dog"
Carolina Cuesta-Lazaro IAIFI/MIT - From Zero to Generative
The building blocks: 3. Loss function

Image Credit: "Visualizing the loss landscape of neural networks" Hao Li et al
Carolina Cuesta-Lazaro IAIFI/MIT - From Zero to Generative

The building blocks: 4. The Optimizer
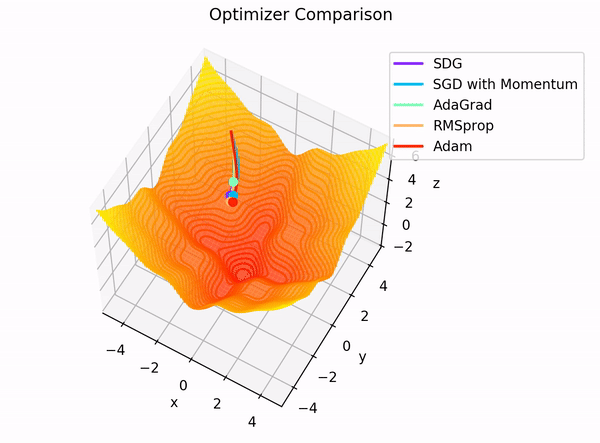
Image Credit: "Complete guide to Adam optimization" Hao Li et al
Carolina Cuesta-Lazaro IAIFI/MIT - From Zero to Generative
Tutorial 1: Learning to classify

Carolina Cuesta-Lazaro IAIFI/MIT - From Zero to Generative
How do we output a probability?
Carolina Cuesta-Lazaro IAIFI/MIT - From Zero to Generative

Pixel 1
Pixel 2
Pixel N

p Class 1
p Class 2
p Class 10
Loss function: Cross entropy
How different are two probability distributions?


Model Prediction
if True class is for i
otherwise
Carolina Cuesta-Lazaro IAIFI/MIT - From Zero to Generative
Truth: Class = 0
True class
Predicted probability

import flax.linen as nn
class MLP(nn.Module):
@nn.compact
def __call__(self, x):
# Linear
x = nn.Dense(features=64)(x)
# Non-linearity
x = nn.silu(x)
# Linear
x = nn.Dense(features=64)(x)
# Non-linearity
x = nn.silu(x)
# Linear
x = nn.Dense(features=2)(x)
return x
model = MLP()
Jax Models
import jax.numpy as jnp
example_input = jnp.ones((1,4))
params = model.init(jax.random.PRNGKey(0), example_input)
y = model.apply(params, example_input)
Architecture
Parameters
Call

Generation vs Discrimination
Carolina Cuesta-Lazaro IAIFI/MIT - From Zero to Generative
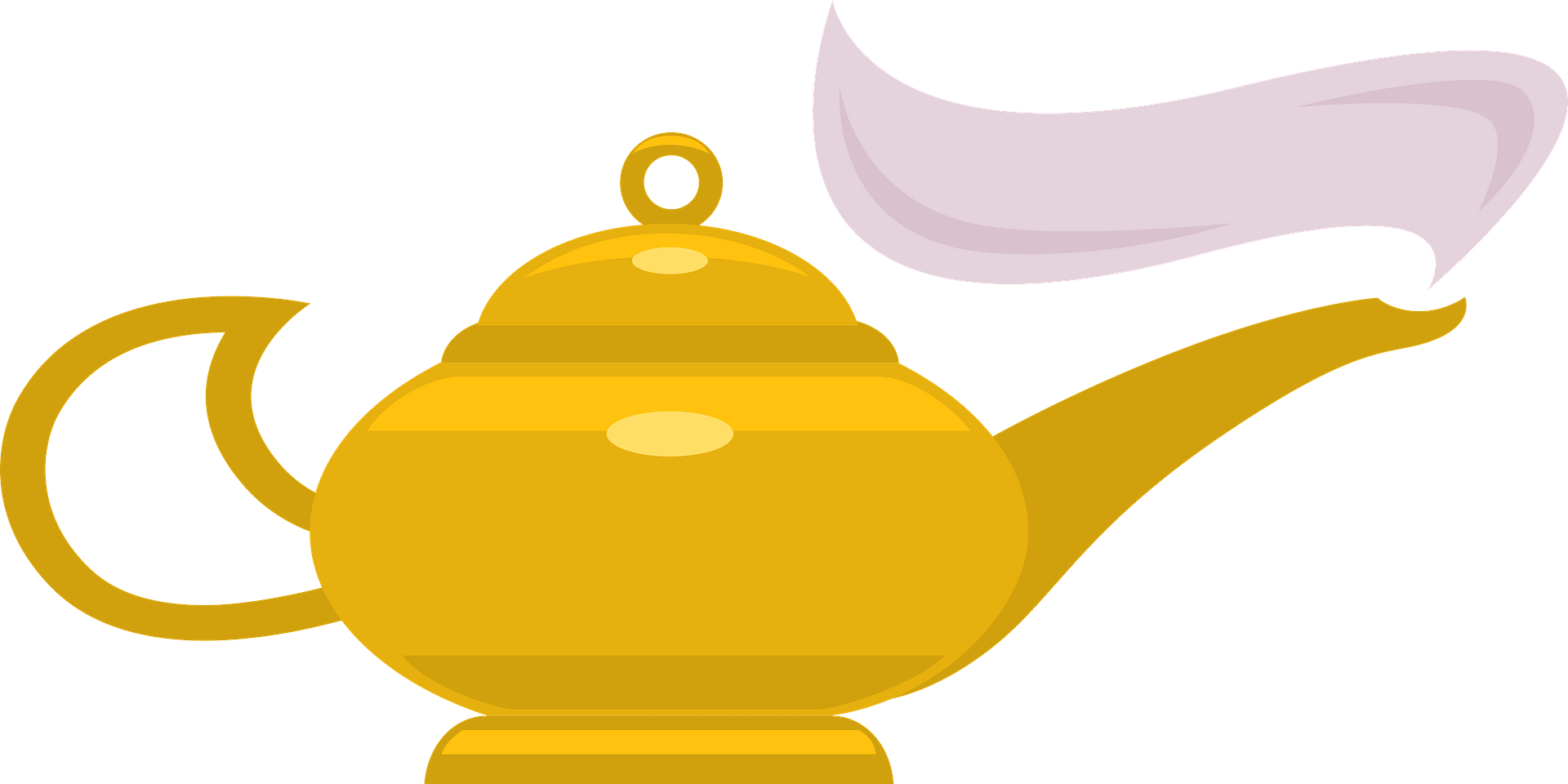
Data
A PDF that we can optimize
Maximize the likelihood of the data
Generative Models
Carolina Cuesta-Lazaro IAIFI/MIT - From Zero to Generative
Generative Models 101
Maximize the likelihood of the training samples
Parametric Model


Training Samples
Carolina Cuesta-Lazaro IAIFI/MIT - From Zero to Generative
Trained Model

Evaluate probabilities


Low Probability
High Probability

Generate Novel Samples


Simulator
Generative Model
Generative Model
Simulator
Generative Models: Simulate and Analyze
Carolina Cuesta-Lazaro IAIFI/MIT - From Zero to Generative
The Generative Zoo
Carolina Cuesta-Lazaro IAIFI/MIT - From Zero to Generative

GANS
VAEs
Normalizing
Flows
Diffusion Models
[Image Credit: https://lilianweng.github.io/posts/2018-10-13-flow-models/]
Bridging two distributions

Base
Data
How is the bridge constrained?
Normalizing flows: Reverse = Forward inverse
Diffusion: Forward = Gaussian noising
Flow Matching: Forward = Interpolant
is p(x0) restricted?
Diffusion: p(x0) is Gaussian
Normalising flows: p(x0) can be evaluated
Is bridge stochastic (SDE) or deterministic (ODE)?
Diffusion: Stochastic (SDE)
Normalising flows: Deterministic (ODE)
Change of variables
sampled from a Gaussian distribution with mean 0 and variance 1
How is
distributed?
Base distribution

Target distribution


Invertible transformation
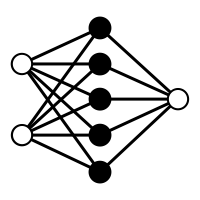
Normalizing flows
Carolina Cuesta-Lazaro IAIFI/MIT - From Zero to Generative


Box-Muller transform
Normalizing flows in 1934
Carolina Cuesta-Lazaro IAIFI/MIT - From Zero to Generative
Normalizing flows
[Image Credit: "Understanding Deep Learning" Simon J.D. Prince]
Bijective
Sample
Evaluate probabilities
Probability mass conserved locally
Carolina Cuesta-Lazaro IAIFI/MIT - From Zero to Generative
Image Credit: "Understanding Deep Learning" Simon J.D. Prince
Carolina Cuesta-Lazaro IAIFI/MIT - From Zero to Generative

Invertible functions aren't that common!
Splines
Carolina Cuesta-Lazaro IAIFI/MIT - From Zero to Generative
Issues NFs: Lack of flexibility
- Invertible functions
- Tractable Jacobians
Masked Autoregressive Flows
Neural Network


Sample
Evaluate probabilities
Carolina Cuesta-Lazaro IAIFI/MIT - From Zero to Generative
Forward Model
Observable
Dark matter
Dark energy
Inflation
Predict
Infer

Parameters
Inverse mapping

Fault line stress
Plate velocity

Carolina Cuesta-Lazaro IAIFI/MIT - From Zero to Generative
Simulation-based Inference
Normalizing flow
In continuous time
Continuity Equation
[Image Credit: "Understanding Deep Learning" Simon J.D. Prince]
Carolina Cuesta-Lazaro IAIFI/MIT - From Zero to Generative


Chen et al. (2018), Grathwohl et al. (2018)

Generate
Carolina Cuesta-Lazaro IAIFI/MIT - From Zero to Generative
Evaluate Probability
Carolina Cuesta-Lazaro IAIFI/MIT - From Zero to Generative
Loss requires solving an ODE!
Diffusion, Flow matching, Interpolants... All ways to avoid this at training time
Conditional Flow matching
Assume a conditional vector field (known at training time)
The loss that we can compute
The gradients of the losses are the same!

["Flow Matching for Generative Modeling" Lipman et al]
["Stochastic Interpolants: A Unifying framework for Flows and Diffusions" Albergo et al]
Intractable
Carolina Cuesta-Lazaro IAIFI/MIT - From Zero to Generative
Flow Matching
Continuity equation
[Image Credit: "Understanding Deep Learning" Simon J.D. Prince]
Sample
Evaluate probabilities
Carolina Cuesta-Lazaro IAIFI/MIT - From Zero to Generative
Diffusion Models
Reverse diffusion: Denoise previous step
Forward diffusion: Add Gaussian noise (fixed)




Prompt
A person half Yoda half Gandalf
Denoising = Regression
Fixed base distribution:
Gaussian
Carolina Cuesta-Lazaro IAIFI/MIT - From Zero to Generative

["A point cloud approach to generative modeling for galaxy surveys at the field level"
Cuesta-Lazaro and Mishra-Sharma
International Conference on Machine Learning ICML AI4Astro 2023, Spotlight talk, arXiv:2311.17141]
Base Distribution
Target Distribution
Simulated Galaxy 3d Map
Prompt:




Prompt: A person half Yoda half Gandalf
Carolina Cuesta-Lazaro IAIFI/MIT - From Zero to Generative
Tutorial 2

Gaussian
MNIST
Carolina Cuesta-Lazaro IAIFI/MIT - From Zero to Generative
Students at MIT are
Pre-trained on next word prediction
...
OVER-CAFFEINATED
NERDS
SMART
ATHLETIC
Large Language Models
Carolina Cuesta-Lazaro IAIFI/MIT - From Zero to Generative
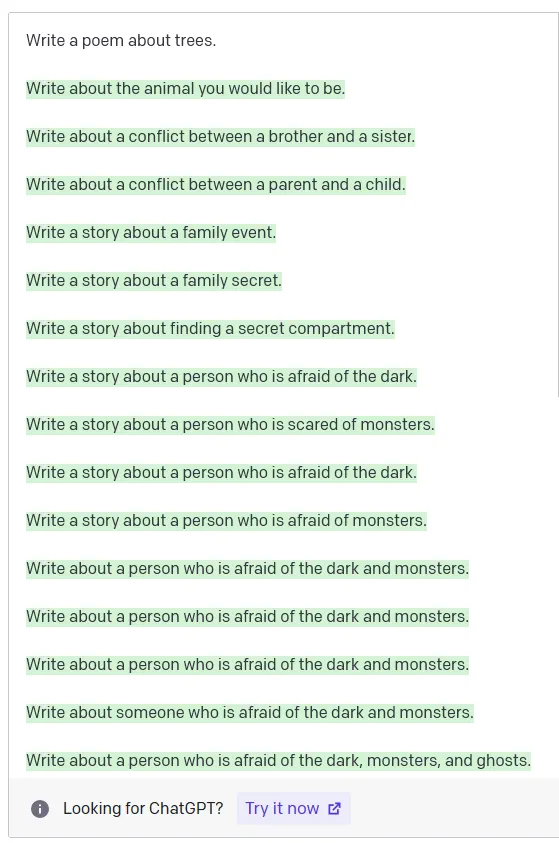
https://www.astralcodexten.com/p/janus-simulators
How do we encode "helpful" in the loss function?
Carolina Cuesta-Lazaro IAIFI/MIT - From Zero to Generative
Step 1
Human teaches desired output
Explain RLHF

After training the model...
Step 2
Human scores outputs
+ teaches Reward model to score
it is the method by which ...
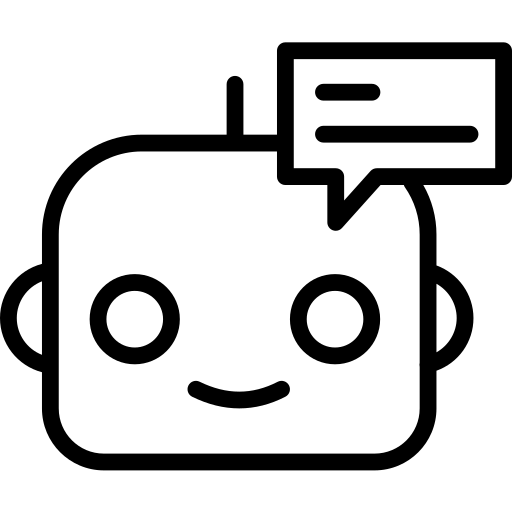
Explain means to tell someone...
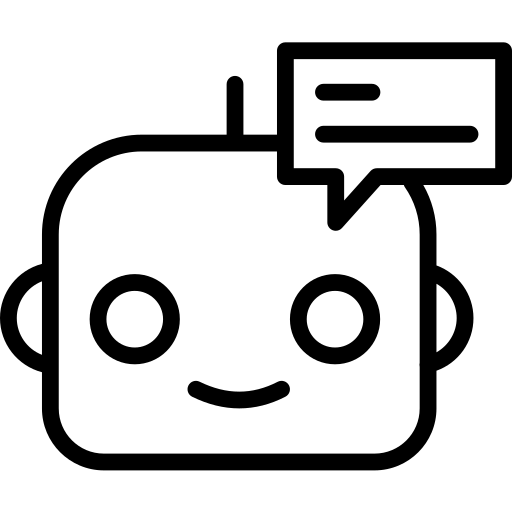
Explain RLHF
Step 3
Tune the Language Model to produce high rewards!
RLHF: Reinforcement Learning from Human Feedback
Carolina Cuesta-Lazaro IAIFI/MIT - From Zero to Generative
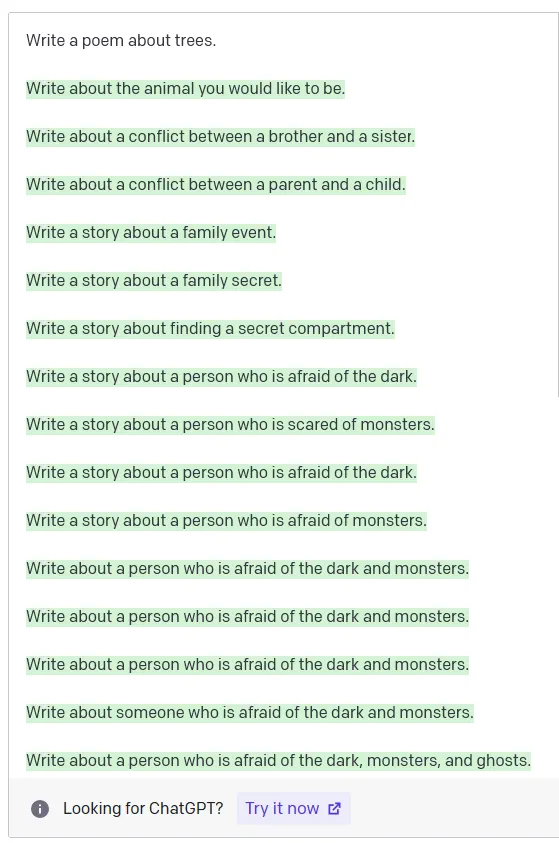
BEFORE RLHF
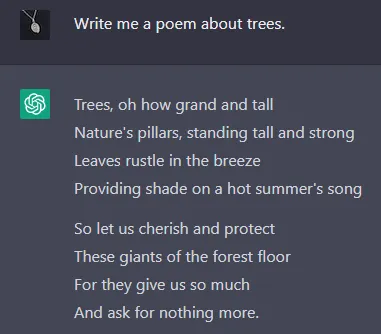
AFTER RLHF
Carolina Cuesta-Lazaro IAIFI/MIT - From Zero to Generative

Carolina Cuesta-Lazaro IAIFI/MIT - From Zero to Generative
Reasoning
Carolina Cuesta-Lazaro IAIFI/MIT - From Zero to Generative
Reasoning

Carolina Cuesta-Lazaro IAIFI/MIT - From Zero to Generative
RLVR (Verifiable Rewards)

Examples: Code execution, game playing, instruction following ....
[Image Credit: AgentBench https://arxiv.org/abs/2308.03688]
Carolina Cuesta-Lazaro IAIFI/MIT - From Zero to Generative
Agents

-
Books by Kevin P. Murphy
- Machine learning, a probabilistic perspective
- Probabilistic Machine Learning: advanced topics
- ML4Astro workshop https://ml4astro.github.io/icml2023/
- ProbAI summer school https://github.com/probabilisticai/probai-2023
- IAIFI Summer school
- Blogposts
Carolina Cuesta-Lazaro IAIFI/MIT - From Zero to Generative
References
cuestalz@mit.edu

From zero to generative - Arizona - 2025
By carol cuesta
From zero to generative - Arizona - 2025
- 180